The Impact of AI-Generated Content on LLM Training and the Internet: A Double-Edged Sword
Written By Chinmay Kapoor
Date Posted June 21st, 2024
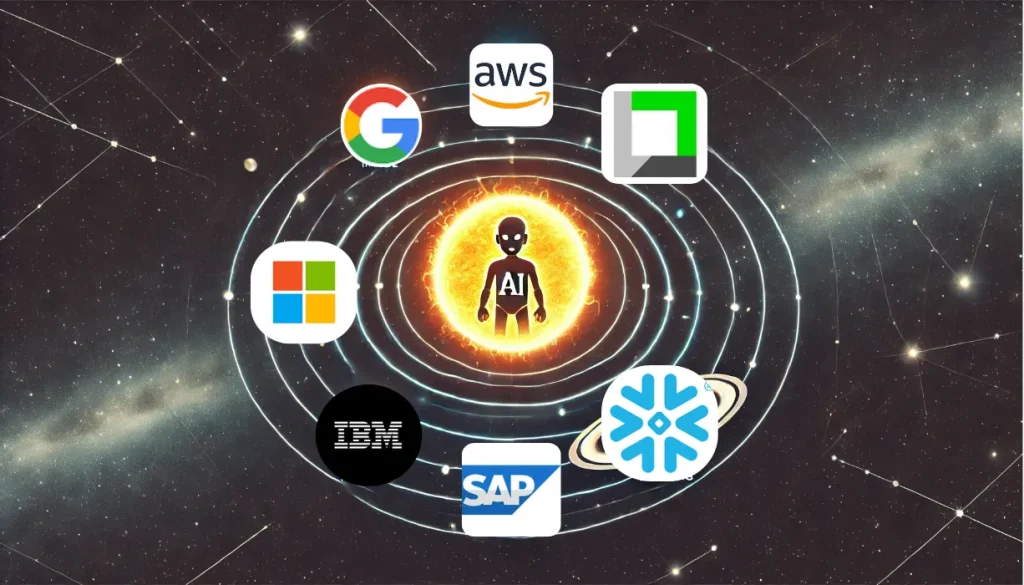
Introduction
The rapid advancement of artificial intelligence has brought about significant changes in various domains, particularly in natural language processing. Large language models (LLMs) like GPT-3 and GPT-4 have shown remarkable capabilities in generating human-like text. However, the proliferation of AI-generated content on the internet poses critical questions about its impact on the correctness and reliability of these models. This blog explores the implications of using AI-generated content as a testing dataset for continuous training of LLMs, the current and future state of AI-generated content on the internet, and whether we should be concerned.
The Current Landscape
AI-Generated Content on the Internet
AI-generated content is becoming increasingly prevalent across the internet. From automated news articles to social media posts and creative writing, AI’s footprint is expanding. While exact figures are difficult to pinpoint, estimates suggest that AI-generated content could account for approximately 10–15% of the internet’s textual content. This percentage is projected to grow as AI technology continues to improve and become more accessible. Predictions indicate that by 2030, AI-generated content could comprise over 50% of the internet’s textual data, with some reports suggesting it could reach as high as 90% by 2026.
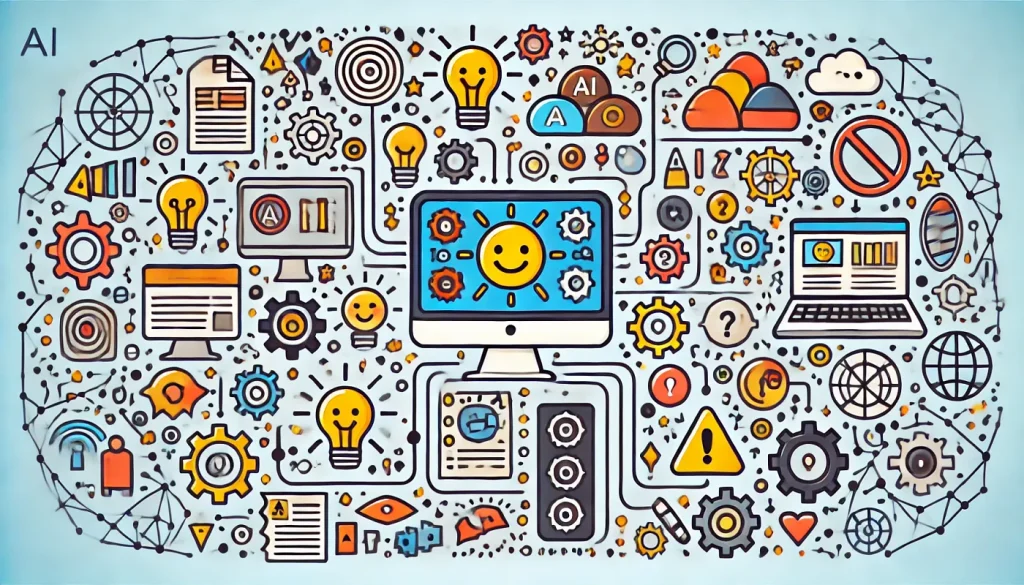
Source: CHAT GPT 4o, Dall-e
Public Perception of AI
A recent survey by Public First highlights the mixed emotions surrounding the rise of AI. While curiosity is the most common reaction, there is also significant excitement and worry. Notably, 40% of current workers believe that AI could outperform them in their jobs within the next decade, and 64% expect AI to significantly increase unemployment. These findings underscore the need for a nuanced approach to integrating AI into our digital and professional lives.
Continuous Training of LLMs
Correctness and Reliability
Using AI-generated content as training data for LLMs poses unique challenges. While the abundance of AI-generated content can provide a vast amount of data, it also risks reinforcing errors and biases inherent in synthetic media. Over-reliance on AI-generated content can lead to a homogenized dataset, limiting the model’s ability to generalize and potentially degrading its correctness ratio.
Bias Propagation
AI-generated content often reflects the biases present in its training data. If this content is fed back into LLMs for continuous training, it can perpetuate and amplify these biases, leading to models that are less reliable and more prone to generating biased or inaccurate outputs. This cyclical reinforcement of bias underscores the importance of maintaining diverse and representative training datasets.
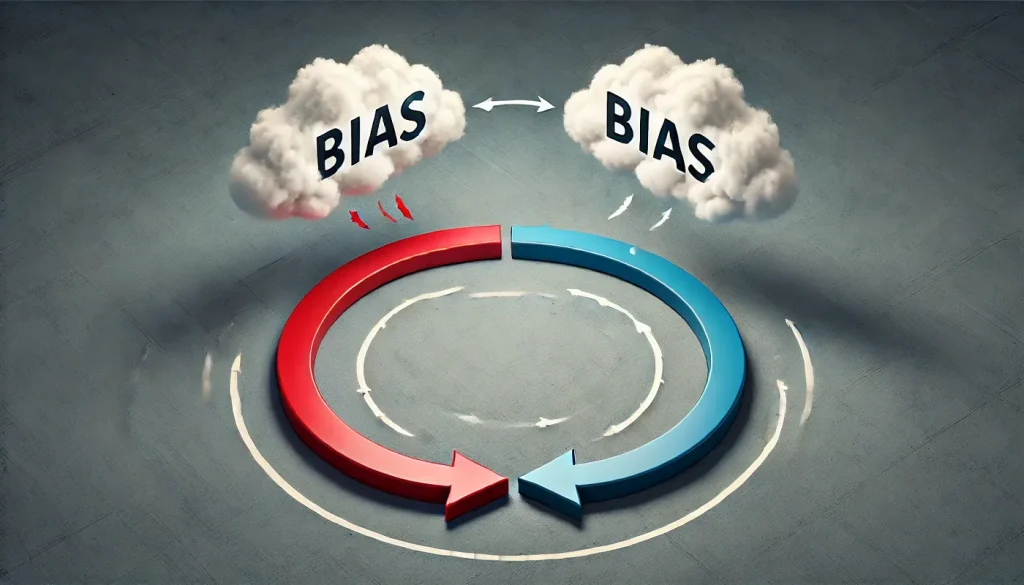
Source: CHAT GPT 4o, Dall-e
The Broader Impact on Digital Content
Echo Chambers and Filter Bubbles
The proliferation of AI-generated content can exacerbate the issue of echo chambers and filter bubbles, where users are increasingly exposed to information that reinforces their pre-existing beliefs. This dynamic can polarize public discourse, erode trust in legitimate news sources, and diminish critical engagement.
The Anti-Content Movement
As AI-generated content becomes more prevalent, the human element in digital interactions will become a coveted rarity. The Anti-Content Movement, inspired by this trend, emphasizes the value of authentic, human-generated content. This movement highlights the importance of distinguishing genuine human interaction from AI-generated facades, fostering a digital environment where the nuances of human communication are celebrated.
Strategies for Mitigating Risks
Hybrid Datasets:
To mitigate the risks associated with AI-generated content, it is crucial to use hybrid datasets that combine AI-generated and human-generated content. This approach ensures a balanced and representative training dataset, helping LLMs maintain their ability to generalize across diverse contexts.
Bias Detection & Mitigation:
Developers should prioritize ethical considerations in AI development, ensuring that models are designed to minimize biases and errors. This involves incorporating fairness and bias detection frameworks during the training phase. Research from PNAS suggests that AI systems trained with bias detection mechanisms can reduce discriminatory outcomes by up to 40%.
Algorithmic Fairness: Implementing techniques to detect and mitigate biases in AI-generated content is crucial to prevent the propagation of these biases in the models. Algorithms such as re-weighting, re-sampling, and adversarial debiasing can be employed to address biases in training data. Research published in the Journal of Machine Learning Research indicates that these techniques can reduce bias by up to 50% in certain applications.
Regular Audits: Conducting regular audits of AI systems can help identify and address potential biases. Audits involve systematically evaluating the model’s outputs and underlying data to detect bias and ensure fairness. A report by AI Now Institute recommends annual audits for high-stakes AI systems to ensure ongoing compliance with ethical standards.
Transparency
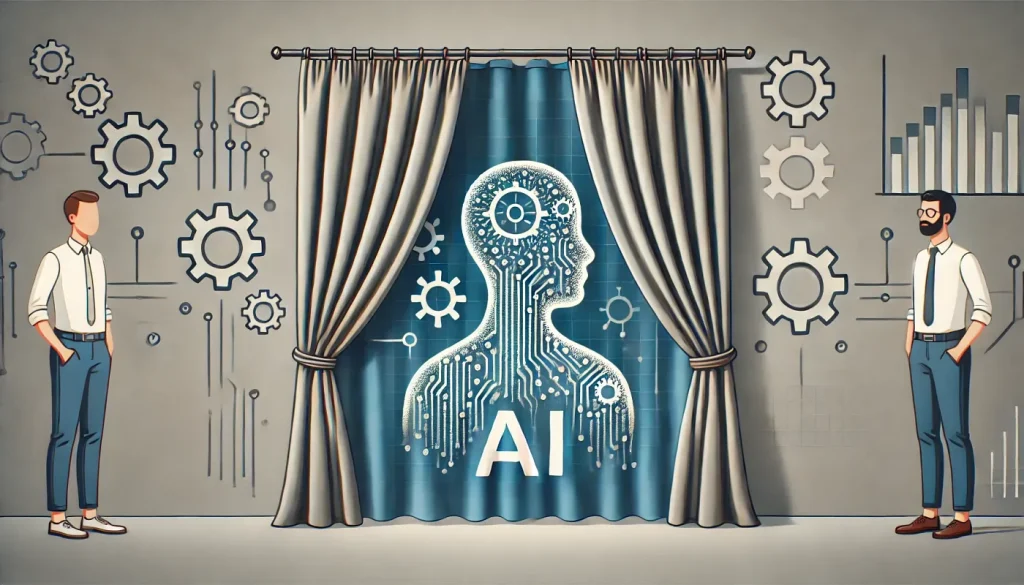
Clear Labeling: Platforms should clearly label AI-generated content to distinguish it from human-generated text. This transparency allows users to make informed judgments about the information they consume. According to a 2023 survey by Public First, 76% of consumers are concerned about misinformation from AI, and only 56% believe they can tell the difference between AI-generated and human-generated content. Clear labeling can help bridge this gap and enhance user trust.
Disclosure Standards: Setting industry-wide standards for disclosure of AI-generated content can further improve transparency. For instance, the European Union’s AI Act proposes mandatory disclosures for AI-generated content, aiming to address the growing concerns about misinformation and synthetic media.
Quality Control
Automated Filtering: Implementing robust quality control mechanisms can help identify and filter out low-quality or erroneous AI-generated content. Automated filtering systems, enhanced with machine learning algorithms, can detect inconsistencies, factual errors, and low-quality outputs. For example, Facebook’s AI-driven content moderation tools reportedly detect and remove over 95% of hate speech before it is reported by users.
Human Oversight: Despite the advancements in automated systems, human oversight remains crucial. Combining AI tools with human review can improve accuracy and reliability. According to the MIT Technology Review, human moderators working alongside AI can reduce the error rate of content moderation systems by up to 50%.
The Role of Data Centers and Archiving
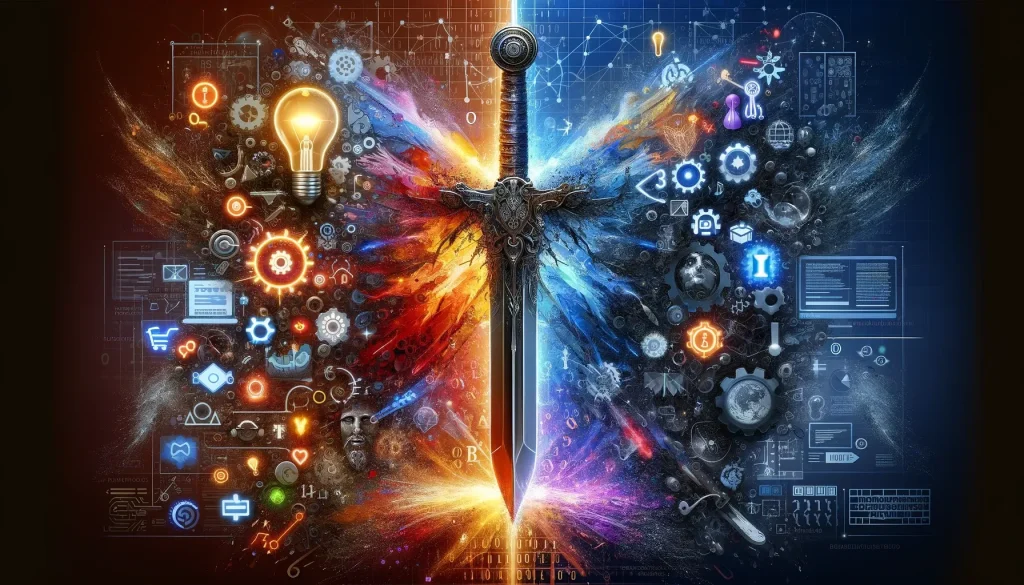
Data Storage and Management
The exponential growth of AI-generated content necessitates efficient data storage and management solutions. Data centers will play a crucial role in accommodating the increasing volume of digital content, ensuring it is stored, archived, and accessible for future use.
Growth in Data Volume: According to a report by IDC, the global datasphere is expected to grow from 33 zettabytes in 2018 to 175 zettabytes by 2025. A significant portion of this growth will be driven by AI-generated content. With AI-generated content projected to comprise up to 90% of online data by 2026, the demand for scalable storage solutions is unprecedented.
Scalable Infrastructure: Data centers must be equipped with scalable infrastructure to handle the surging data volumes. This involves adopting advanced storage technologies such as NVMe (Non-Volatile Memory Express) and SSDs (Solid State Drives), which offer higher performance and reliability compared to traditional HDDs (Hard Disk Drives). For instance, NVMe storage solutions can provide up to 6x faster data access speeds, which is critical for managing large datasets efficiently.
Energy Efficiency: As data centers expand to accommodate more data, energy consumption becomes a significant concern. Implementing energy-efficient practices is crucial. Modern data centers are increasingly using renewable energy sources, such as solar and wind power, to reduce their carbon footprint. For example, Google has committed to operating its data centers on 100% renewable energy, achieving significant reductions in their environmental impact.
Data Security: Ensuring the security of stored data is paramount. Data centers employ advanced encryption techniques and secure access protocols to protect sensitive information. Technologies like homomorphic encryption, which allows data to be processed without being decrypted, are being explored to enhance data security further. According to a survey by Cybersecurity Ventures, the global spending on cybersecurity solutions is expected to exceed $1 trillion from 2017 to 2021, reflecting the importance of securing data in modern storage facilities.
Data Archiving and Retrieval
Effective data archiving methods are essential for preserving the integrity of digital content. This includes developing technologies to detect and archive AI-generated content separately from human-generated content, ensuring that historical data remains reliable and verifiable.
Archiving AI-Generated Content: One of the key challenges in archiving AI-generated content is distinguishing it from human-generated content. Advanced AI algorithms can be employed to detect synthetic media by analyzing patterns and anomalies in the data. For example, machine learning models trained on large datasets can identify subtle inconsistencies that are characteristic of AI-generated content.
Separate Archiving: Storing AI-generated content separately from human-generated content ensures the integrity of historical data. This separation can prevent the unintentional blending of synthetic and authentic data, which is crucial for future data analysis and research. Separate archiving also facilitates easier retrieval and verification of content, ensuring that users can access the original, unaltered data when needed.
Long-term Storage Solutions: Long-term data archiving requires storage solutions that can preserve data integrity over extended periods. Technologies like magnetic tape storage, which offers a lifespan of up to 30 years, are commonly used for archival purposes. According to the LTO (Linear Tape-Open) Consortium, LTO tape storage can provide a cost-effective and reliable solution for archiving large volumes of data, with storage capacities reaching up to 30TB per cartridge in the latest LTO-9 generation
Should We Be Afraid?
The potential risks associated with the growing presence of AI-generated content are real, but they can be managed through careful oversight and ethical guidelines. Here are some considerations:
Ethical AI Development
Establishing and adhering to ethical guidelines for AI development can ensure responsible use. Organizations like the Partnership on AI have developed comprehensive frameworks that promote transparency, accountability, and fairness in AI systems. These guidelines can help prevent the propagation of harmful biases and errors. Developers should prioritize ethical considerations in AI development, ensuring that models are designed to minimize biases and errors.
Evolving AI Models
To effectively incorporate AI-generated content into training datasets without compromising model performance, the following strategies should be considered: Evolving AI Models
To effectively incorporate AI-generated content into training datasets without compromising model performance, the following strategies should be considered:
Hybrid Datasets
Diverse Data Sources: Combining AI-generated content with diverse human-generated content can help maintain a balanced and representative training dataset. Studies have shown that hybrid datasets can improve model performance by enhancing the diversity of training examples. For instance, a hybrid dataset approach increased the accuracy of a sentiment analysis model by 15% in a study conducted by Stanford University.
Cross-Validation: Implementing cross-validation techniques can ensure that models trained on hybrid datasets generalize well across different contexts. This approach involves partitioning the dataset into multiple subsets and training the model on each subset iteratively. Cross-validation has been shown to improve model robustness and reduce overfitting by 20–30%
Continuous Monitoring
Performance Evaluation: Regularly evaluating the performance and correctness of models trained with AI-generated content can help identify and address potential issues early on. Performance metrics such as accuracy, precision, recall, and F1 score should be continuously monitored. For example, Google’s AI team implements continuous monitoring of their models, leading to a 25% reduction in deployment errors.
Feedback Loops: Establishing feedback loops that incorporate user feedback and error analysis can enhance model performance. User feedback can provide valuable insights into the model’s strengths and weaknesses, enabling iterative improvements. According to a study by Microsoft Research, incorporating user feedback into the training loop can improve model accuracy by 10–15%.
Conclusion
The increasing presence of AI-generated content on the internet presents both opportunities and challenges. While synthetic media can enrich training datasets and provide diverse textual styles, it also risks reinforcing biases and diminishing the correctness of LLMs. To navigate this complex landscape, it is essential to adopt strategies that maintain the integrity of online content, foster human-centric digital practices, and ensure robust data management solutions. By doing so, we can harness the power of AI-generated content while preserving the authenticity and reliability of the digital world.